Uncategorized
A Response To A Question About Post-Keynesian Interest Rate Theories (…And A Rant)
I got a question about references for post-Keynesian theories of interest rates. My answer to this has a lot of levels, and eventually turns into a rant about modern academia. Since I do not want a good rant to go to waste, I will spell it out here. Lo…
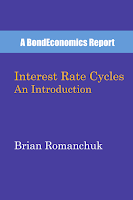
I got a question about references for post-Keynesian theories of interest rates. My answer to this has a lot of levels, and eventually turns into a rant about modern academia. Since I do not want a good rant to go to waste, I will spell it out here. Long-time readers may have seen portions of this rant before, but my excuse is that I have a lot of new readers.
(I guess I can put a plug in for my book Interest Rate Cycles: An Introduction which covers a variety of topics around interest rates.)
References (Books)
For reasons that will become apparent later, I do not have particular favourites among post-Keynesian theories of interest rates. For finding references, I would give the following three textbooks as starting points. Note that as academic books, they are pricey. However, starting with textbooks is more efficient from a time perspective than trawling around on the internet looking for free articles.
Mitchell, William, L. Randall Wray, and Martin Watts. Macroeconomics. Bloomsbury Publishing, 2019. Notes: This is an undergraduate-level textbook, and references are very limited. For the person who originally asked the question, this is perhaps not the best fit.
Lavoie, Marc. Post-Keynesian economics: new foundations. Edward Elgar Publishing, 2022. This is an introductory graduate level textbook, and is a survey of PK theory. Chuck full o’ citations.
Godley, Wynne, and Marc Lavoie. Monetary economics: an integrated approach to credit, money, income, production and wealth. Springer, 2006. This book is an introduction to stock-flow consistent models, and is probably the best starting point for post-Keynesian economics for someone with mathematical training.
Why Books?
If you have access to a research library and you have at least some knowledge of the field one can do a literature survey by going nuts chasing down citations from other papers. I did my doctorate in the Stone Age, and I had a stack of several hundred photocopied papers related to my thesis sprawled across on my desk1, and I periodically spent the afternoon camped out in the journal section of the engineering library reading and photocopying the interesting ones.
If you do not have access to such a library, it is painful getting articles. And if you do not know the field, you are making a grave mistake to rely on articles to get a survey of the field.
In order to get published, authors have no choice but to hype up their own work. Ground-breaking papers have cushion to be modest, but more than 90% of academic papers ought never have to been published, so they have no choice but to self-hype.
Academic politics skews what articles are cited. Also, alternative approaches need to be minimised in order handing ammunition to reviewers looking to reject the paper.
The adversarial review process makes it safer to avoid anything remotely controversial in areas of the text that are not part of what allegedly makes it novel.
My points here might sound cynical, but they just are what happens if you apply any minimal intellectual standards to modern academic output. Academics have to churn out papers; the implication is that the papers cannot all be winners.
The advantage of an “introductory post-graduate” textbook is that the author is doing the survey of a field, and it is exceedingly unlikely that they produced all the research being surveyed. This allows them to take a “big picture” view of the field, without worries about originality. Academic textbooks are not cheap, but if you value your time (or have an employer to pick up the tab), they are the best starting point.
My Background
One of my ongoing jobs back when I was an employee was doing donkey work for economists. To the extent that I had any skills, I was a “model builder.” And so I was told to go off and build models according to various specifications.
Over the years, I developed hundreds (possibly thousands) of “reduced order” models that related economic variables to interest rates, based on conventional and somewhat heterodox thinking. (“Reduced order” has a technical definition, I am using the term somewhat loosely. “Relatively simple models with a limited number of inputs and outputs” is what I mean.) Of course, I also mucked around with the data and models on my own initiative.
To summarise my thinking, I am not greatly impressed with the ability of reduced order models to tell us about the effect of interest rates. And it is not hard to prove me wrong — just come up with such a reduced form model. The general lack of agreement on such a model is a rather telling point.
“Conventional” Thinking On Interest Rates
The conventional thinking on interest rates is that if inflation is too high, the central bank hikes the policy rate to slow it. The logic is that if interest rates go up, growth or inflation (or both) falls. This conventional thinking is widespread, and even many post-Keynesians agree with it.
Although there is widespread consensus about that story, the problem shows up as soon as you want to put numbers to it. An interest rate is a number. How high does it have to be to slow inflation?
Back in the 1990s, there were a lot of people who argued that the magic cut off point for the effect of the real interest rate was the “potential trend growth rate” of the economy (working age population growth plus productivity growth). The beauty of that theory is that it gave a testable prediction. The minor oopsie was that it did not in fact work.
So we were stuck with what used to be called the “neutral interest rate” — now denoted r* — which moves around (a lot). The level of r* is estimated with a suitably complex statistical process. The more complex the estimation the better — since you need something to distract from the issue of non-falsifiability. If you use a model to calibrate r* on historical data, by definition your model will “predict” historical data when you compare the actual real policy rate versus your r* estimate. The problem is that if r* keeps moving around — it does not tell you much about the future. For example, is a real interest rate of 2% too low to cause inflation to drop? Well, just set it at 2% and see whether it drops! The problem is that you only find out later.
Post-Keynesian Interest Rate Models
If you read the Marc Lavoie “New Foundations” text, you will see that what defines “post-Keynesian” economics is difficult. There are multiple schools of thought (possibly with some “schools” being one individual) — one of which he labels “Post-Keynesian” (capital P) that does not consider anybody else to be “post-Keynesian.” I take what Lavoie labels as the “broad tent” view, and accept that PK economics is a mix of schools of thought that have some similarities — but still have theoretical disagreements.
Interest rate theories is one of big areas of disagreement. This is especially true after the rise of Modern Monetary Theory (MMT).
MMT is a relatively recent offshoot of the other PK schools of thought. What distinguished MMT is that the founders wanted to come up with an internally consistent story that could be used to convince outsiders to act more sensibly. The effect of interest rates on the economy was one place where MMTers have a major disagreement with others in the broad PK camp, and even the MMTers have somewhat varied positions.
The best way to summarise the consensus MMT position is that the effect of interest rates on the economy are ambiguous, and weaker than conventional beliefs. A key point is that interest payments to the private sector are a form of income, and thus a weak stimulus. (The mainstream awkwardly tries to incorporate this by bringing up “fiscal dominance” — but fiscal dominance is just decried as a bad thing, and is not integrated into other models.) Since this stimulus runs counter to the conventional belief that interest rates slow the economy, one can argue that conventional thinking is backwards. (Warren Mosler emphases this, other MMT proponents lean more towards “ambiguous.”)
For what it is worth, “ambiguous” fits my experience of churning out interest rate models, so that is good enough for me. I think that if we want to dig, we need to look at the interest rate sensitivity of sectors, like housing. The key is that we are not looking at an aggregated model of the economy — once you have multiple sectors, we can get ambiguous effects.
What about the Non-MMT Post-Keynesians?
The fratricidal fights between MMT economists and selected other post-Keynesians (not all, but some influential ones) was mainly fought over the role of floating exchange rates and fiscal policy, but interest rates showed up. Roughly speaking, they all agreed that neoclassicals are wrong about interest rates. Nevertheless, there are divergences on how effective interest rates are.
I would divide the post-Keynesian interest rate literature into a few segments.
Empirical analysis of the effects of interest rates, mainly on fixed investment.
Fairly basic toy models that supposedly tell us about the effect of interest rates. (“Liquidity preference” models that allegedly tell us about bond yields is one example. I think that such models are a waste of time — rate expectations factor into real world fixed income trading.)
Analysis of why conventional thinking about interest rates is incorrect because it does not take into account some factors.
Analysis of who said what about interest rates (particularly Keynes).
From an outsiders standpoint, the problem is that even if the above topics are interesting, the standard mode of exposition is to jumble these areas of interest into a long literary piece. My experience is that unless I am already somewhat familiar with the topics discussed, I could not follow the logical structure of arguments.
The conclusions drawn varied by economist. Some older ones seem indistinguishable from 1960s era Keynesian economists in their reliance on toy models with a couple of supply/demand curves.
I found the strongest part of this literature was the essentially empirical question: do interest rates affect behaviour in the ways suggested by classical/neoclassical models? The rest of the literature is not structured in a way that I am used to, and so I have a harder time discussing it.
Appendix: Academic Dysfunction
I will close with a rant about the state of modern academia, which also explains why I am not particularly happy with wading through relatively recent (post-1970!) journal articles trying to do a literature search. I am repeating old rant contents here, but I am tacking on new material at the end (which is exactly the behaviour I complain about!).
The structural problem with academia was the rise of the “publish or perish” culture. Using quantitative performance metrics — publications, citation counts — was an antidote to the dangers of mediocrity created by “old boy networks” handing out academic posts. The problem is that the quantitative metric changes behaviour (Goodhart’s Law). Everybody wants their faculty to be above average — and sets their target publication counts accordingly.
This turns academic publishing into a game. It was possible to produce good research, but the trick was to get as many articles out of it as possible. Some researchers are able to produce a deluge of good papers — partly because they have done a good job of developing students and colleagues to act as co-authors. Most are only able to get a few ideas out. Rather than forcing people to produce and referee papers that nobody actually wants to read, everybody should just lower expected publication count standards. However, that sounds bad, so here we are. I left academia because spending the rest of my life churning out articles that I know nobody should read was not attractive.
These problems affect all of academia. (One of the advantages of the college system of my grad school is that it drove you to socialise with grad students in other fields, and not just your own group.) The effects are least bad in fields where there are very high publication standards, or the field is vibrant and new, and there is a lot of space for new useful research.
Pure mathematics is (was?) an example of a field with high publication standards. By definition, there are limited applications of the work, so it attracts less funding. There are less positions, and so the members of the field could be very selective. They policed their journals with their wacky notions of “mathematical elegance,” and this was enough to keep people like me out.
In applied fields (engineering, applied mathematics), publications cannot be policed based on “elegance.” Instead, they are supposed to be “useful.” The way the field decided to measure usefulness was to see whether it could attract industrial partners funding the research. Although one might decry the “corporate influence” on academia, it helps keep applied research on track.
The problem is in areas with no recent theoretical successes. There is no longer an objective way to measure a “good” paper. What happens instead is that papers are published on the basis that they continue the framework of what is seen as a “good” paper in the past.
My academic field of expertise was in such an area. I realised that I had to either get into a new research area that had useful applications — or get out (which I did). I have no interest in the rest of economics, but it is safe to say that macroeconomics (across all fields of thought) has had very few theoretical successes for a very long time. Hence, there is little to stop the degeneration of the academic literature.
Neoclassical macroeconomics has the most people publishing in it, and gets the lion share of funding. Their strange attachment to 1960s era optimal control theory means that the publication standards are closest to what I was used to. The beauty of the mathematical approach to publications is that originality is theoretically easy to assess: has anyone proved this theorem before? You can ignore the textual blah-blah-blah and jump straight to the mathematical meat.
Unfortunately, if the models are useless in practice, this methodology just leaves you open to the problem that there is an infinite number of theorems about different models to be proven. Add in a large accepted gap between the textual representations of what a theorem suggests and what the mathematics does, you end up with a field that has an infinite capacity to produce useless models with irrelevant differences between them. And unlike engineering firms that either go bankrupt or dump failed technology research, researchers at central banks tend to fail upwards. Ask yourself this: if the entire corpus of post-1970 macroeconomic research had been ritually burned, would it have really mattered to how the Fed reacted to the COVID pandemic? (“Oh no, crisis! Cut rates! Oops, inflation! Hike rates!”)
More specifically, neoclassicals have an array of “good” simple macro models that they teach and use as “acceptable” models for future papers. However, those “good” models can easily contradict each other. E.g., use an OLG model to “prove” that debt is burden on future generations, use other models to “prove” that “MMT says nothing new.” Until a model is developed that is actually useful, they are doomed to keep adding epicycles to failed models.
Well, if the neoclassical research agenda is borked, that means that the post-Keynesians aren’t, right? Not so fast. The post-Keynesians traded one set of dysfunctions for other ones — they are also trapped in the same publish-or-perish environment. Without mathematical models offering good quantitative predictions about the macroeconomy, it is hard to distinguish “good” literary analysis from “bad” analysis. They are not caught in the trap of generating epicycle papers, but the writing has evolved to be aimed at other post-Keynesians in order to get through the refereeing process. The texts are filled with so many digressions regarding who said what that trying to find a logical flow is difficult.
The MMT literature (that I am familiar with) was cleaner — because it took a '“back to first principles” approach, and focussed on some practical problems. The target audience was fairly explicitly non-MMTers. They were either responding to critiques (mainly post-Keynesians, since neoclassicals have a marked inability to deal with articles not published in their own journals), or outsiders interested in macro issues. It is very easy to predict when things go downhill — as soon as papers are published solely aimed at other MMT proponents.
Is there a way forward? My view is that there is — but nobody wants it to happen. Impossibility theorems could tell us what macroeconomic analysis cannot do. For example, when is it impossible to stabilise an economy with something like a Taylor Rule? In other words, my ideal graduate level macroeconomics theory textbook is a textbook explaining why you cannot do macroeconomic theory (as it is currently conceived).
Driving my German office mate crazy.
Uncategorized
Comments on February Employment Report
The headline jobs number in the February employment report was above expectations; however, December and January payrolls were revised down by 167,000 combined. The participation rate was unchanged, the employment population ratio decreased, and the …
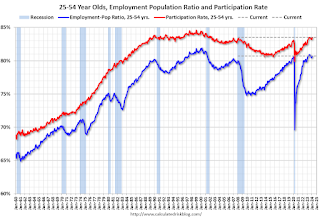
Prime (25 to 54 Years Old) Participation
Since the overall participation rate is impacted by both cyclical (recession) and demographic (aging population, younger people staying in school) reasons, here is the employment-population ratio for the key working age group: 25 to 54 years old.
The 25 to 54 years old participation rate increased in February to 83.5% from 83.3% in January, and the 25 to 54 employment population ratio increased to 80.7% from 80.6% the previous month.
Average Hourly Wages

Wage growth has trended down after peaking at 5.9% YoY in March 2022 and was at 4.3% YoY in February.
Part Time for Economic Reasons

"The number of people employed part time for economic reasons, at 4.4 million, changed little in February. These individuals, who would have preferred full-time employment, were working part time because their hours had been reduced or they were unable to find full-time jobs."The number of persons working part time for economic reasons decreased in February to 4.36 million from 4.42 million in February. This is slightly above pre-pandemic levels.
These workers are included in the alternate measure of labor underutilization (U-6) that increased to 7.3% from 7.2% in the previous month. This is down from the record high in April 2020 of 23.0% and up from the lowest level on record (seasonally adjusted) in December 2022 (6.5%). (This series started in 1994). This measure is above the 7.0% level in February 2020 (pre-pandemic).
Unemployed over 26 Weeks

According to the BLS, there are 1.203 million workers who have been unemployed for more than 26 weeks and still want a job, down from 1.277 million the previous month.
This is close to pre-pandemic levels.
Job Streak
Headline Jobs, Top 10 Streaks | ||
---|---|---|
Year Ended | Streak, Months | |
1 | 2019 | 100 |
2 | 1990 | 48 |
3 | 2007 | 46 |
4 | 1979 | 45 |
5 | 20241 | 38 |
6 tie | 1943 | 33 |
6 tie | 1986 | 33 |
6 tie | 2000 | 33 |
9 | 1967 | 29 |
10 | 1995 | 25 |
1Currrent Streak |
Summary:
The headline monthly jobs number was above consensus expectations; however, December and January payrolls were revised down by 167,000 combined. The participation rate was unchanged, the employment population ratio decreased, and the unemployment rate was increased to 3.9%. Another solid report.
Uncategorized
Immune cells can adapt to invading pathogens, deciding whether to fight now or prepare for the next battle
When faced with a threat, T cells have the decision-making flexibility to both clear out the pathogen now and ready themselves for a future encounter.
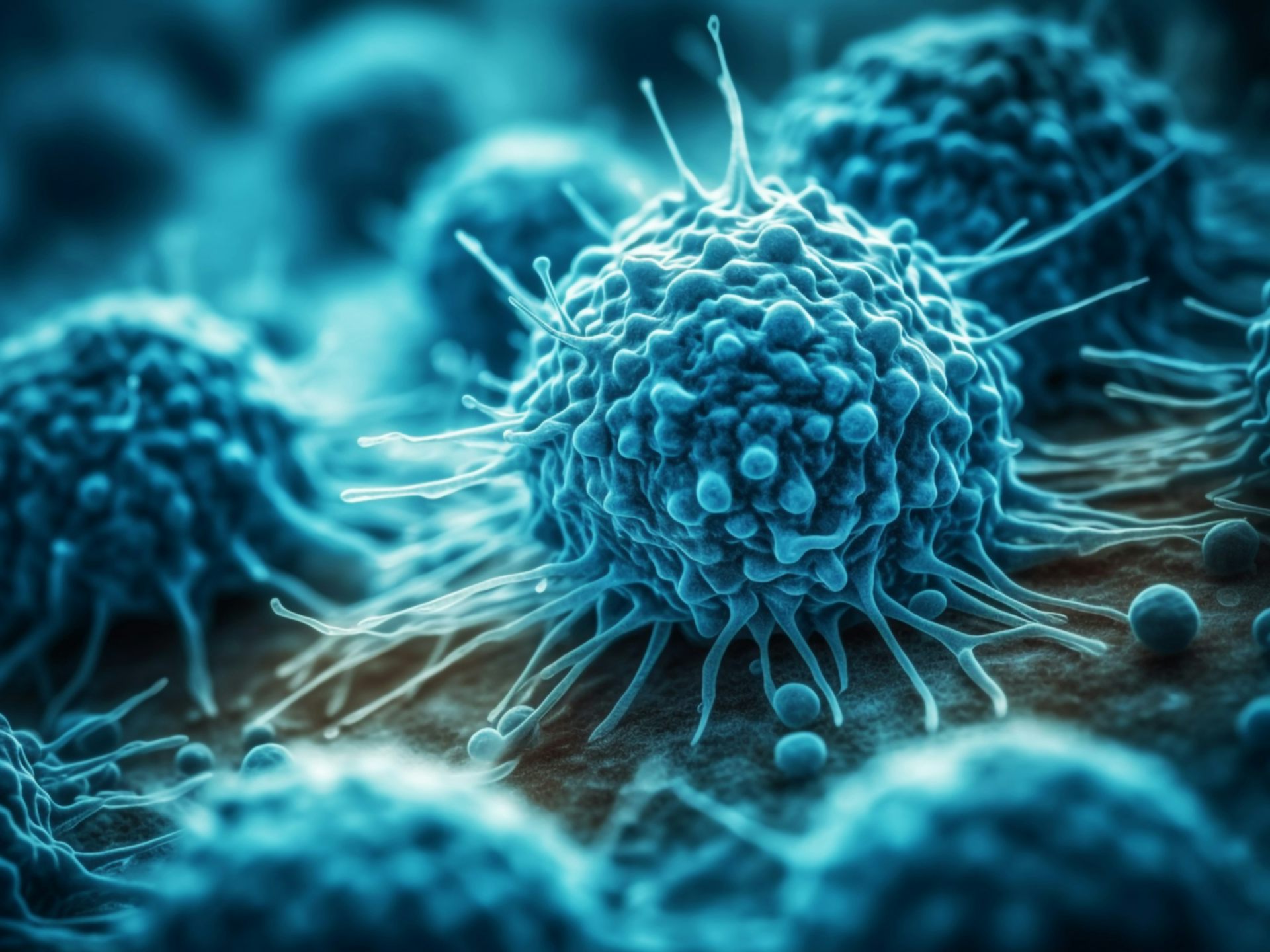
How does your immune system decide between fighting invading pathogens now or preparing to fight them in the future? Turns out, it can change its mind.
Every person has 10 million to 100 million unique T cells that have a critical job in the immune system: patrolling the body for invading pathogens or cancerous cells to eliminate. Each of these T cells has a unique receptor that allows it to recognize foreign proteins on the surface of infected or cancerous cells. When the right T cell encounters the right protein, it rapidly forms many copies of itself to destroy the offending pathogen.

Importantly, this process of proliferation gives rise to both short-lived effector T cells that shut down the immediate pathogen attack and long-lived memory T cells that provide protection against future attacks. But how do T cells decide whether to form cells that kill pathogens now or protect against future infections?
We are a team of bioengineers studying how immune cells mature. In our recently published research, we found that having multiple pathways to decide whether to kill pathogens now or prepare for future invaders boosts the immune system’s ability to effectively respond to different types of challenges.
Fight or remember?
To understand when and how T cells decide to become effector cells that kill pathogens or memory cells that prepare for future infections, we took movies of T cells dividing in response to a stimulus mimicking an encounter with a pathogen.
Specifically, we tracked the activity of a gene called T cell factor 1, or TCF1. This gene is essential for the longevity of memory cells. We found that stochastic, or probabilistic, silencing of the TCF1 gene when cells confront invading pathogens and inflammation drives an early decision between whether T cells become effector or memory cells. Exposure to higher levels of pathogens or inflammation increases the probability of forming effector cells.
Surprisingly, though, we found that some effector cells that had turned off TCF1 early on were able to turn it back on after clearing the pathogen, later becoming memory cells.
Through mathematical modeling, we determined that this flexibility in decision making among memory T cells is critical to generating the right number of cells that respond immediately and cells that prepare for the future, appropriate to the severity of the infection.
Understanding immune memory
The proper formation of persistent, long-lived T cell memory is critical to a person’s ability to fend off diseases ranging from the common cold to COVID-19 to cancer.
From a social and cognitive science perspective, flexibility allows people to adapt and respond optimally to uncertain and dynamic environments. Similarly, for immune cells responding to a pathogen, flexibility in decision making around whether to become memory cells may enable greater responsiveness to an evolving immune challenge.
Memory cells can be subclassified into different types with distinct features and roles in protective immunity. It’s possible that the pathway where memory cells diverge from effector cells early on and the pathway where memory cells form from effector cells later on give rise to particular subtypes of memory cells.
Our study focuses on T cell memory in the context of acute infections the immune system can successfully clear in days, such as cold, the flu or food poisoning. In contrast, chronic conditions such as HIV and cancer require persistent immune responses; long-lived, memory-like cells are critical for this persistence. Our team is investigating whether flexible memory decision making also applies to chronic conditions and whether we can leverage that flexibility to improve cancer immunotherapy.
Resolving uncertainty surrounding how and when memory cells form could help improve vaccine design and therapies that boost the immune system’s ability to provide long-term protection against diverse infectious diseases.
Kathleen Abadie was funded by a NSF (National Science Foundation) Graduate Research Fellowships. She performed this research in affiliation with the University of Washington Department of Bioengineering.
Elisa Clark performed her research in affiliation with the University of Washington (UW) Department of Bioengineering and was funded by a National Science Foundation Graduate Research Fellowship (NSF-GRFP) and by a predoctoral fellowship through the UW Institute for Stem Cell and Regenerative Medicine (ISCRM).
Hao Yuan Kueh receives funding from the National Institutes of Health.
stimulus covid-19 yuan vaccine stimulusUncategorized
Stock indexes are breaking records and crossing milestones – making many investors feel wealthier
The S&P 500 topped 5,000 on Feb. 9, 2024, for the first time. The Dow Jones Industrial Average will probably hit a new big round number soon t…
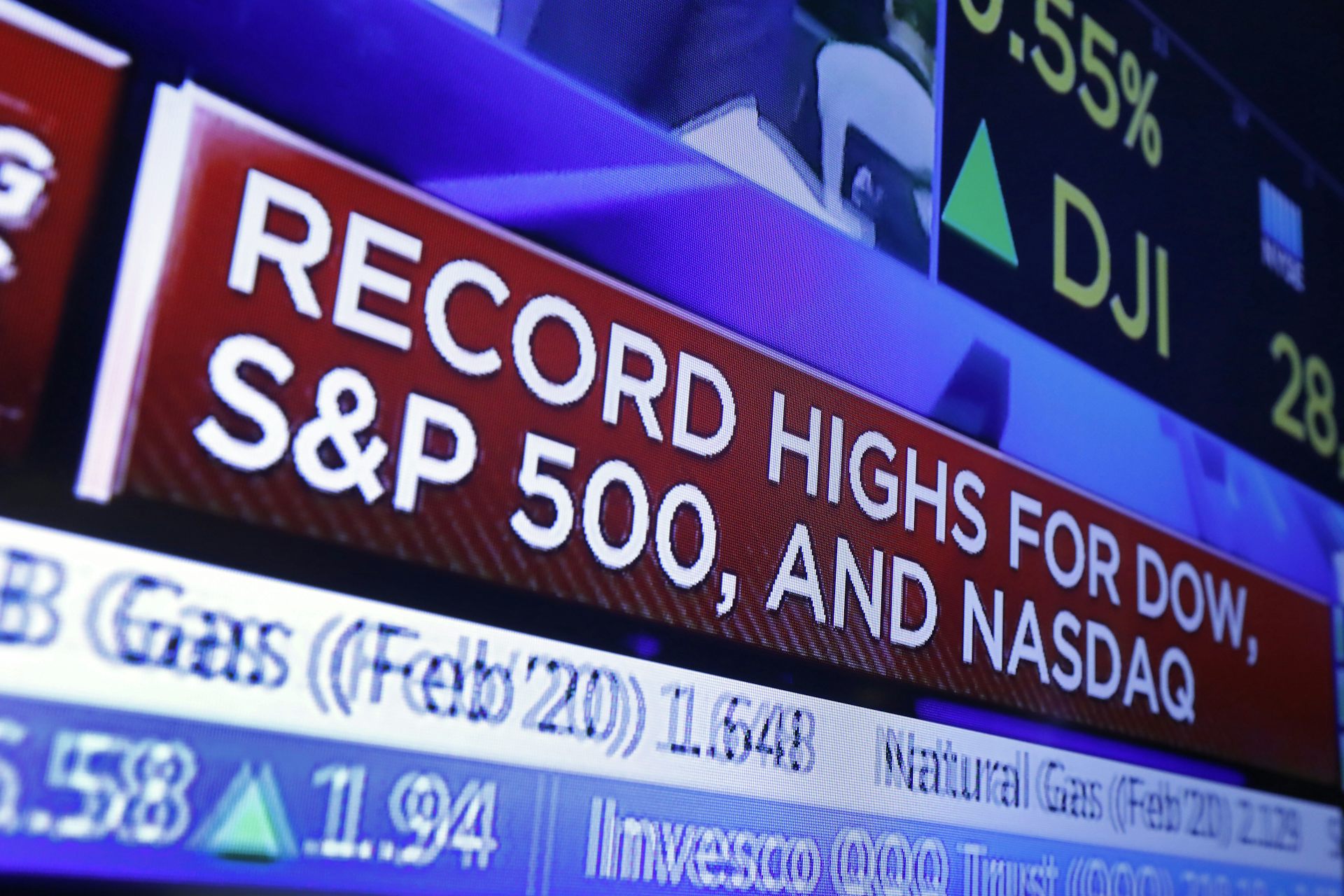
The S&P 500 stock index topped 5,000 for the first time on Feb. 9, 2024, exciting some investors and garnering a flurry of media coverage. The Conversation asked Alexander Kurov, a financial markets scholar, to explain what stock indexes are and to say whether this kind of milestone is a big deal or not.
What are stock indexes?
Stock indexes measure the performance of a group of stocks. When prices rise or fall overall for the shares of those companies, so do stock indexes. The number of stocks in those baskets varies, as does the system for how this mix of shares gets updated.
The Dow Jones Industrial Average, also known as the Dow, includes shares in the 30 U.S. companies with the largest market capitalization – meaning the total value of all the stock belonging to shareholders. That list currently spans companies from Apple to Walt Disney Co.
The S&P 500 tracks shares in 500 of the largest U.S. publicly traded companies.
The Nasdaq composite tracks performance of more than 2,500 stocks listed on the Nasdaq stock exchange.
The DJIA, launched on May 26, 1896, is the oldest of these three popular indexes, and it was one of the first established.
Two enterprising journalists, Charles H. Dow and Edward Jones, had created a different index tied to the railroad industry a dozen years earlier. Most of the 12 stocks the DJIA originally included wouldn’t ring many bells today, such as Chicago Gas and National Lead. But one company that only got booted in 2018 had stayed on the list for 120 years: General Electric.
The S&P 500 index was introduced in 1957 because many investors wanted an option that was more representative of the overall U.S. stock market. The Nasdaq composite was launched in 1971.
You can buy shares in an index fund that mirrors a particular index. This approach can diversify your investments and make them less prone to big losses.
Index funds, which have only existed since Vanguard Group founder John Bogle launched the first one in 1976, now hold trillions of dollars .
Why are there so many?
There are hundreds of stock indexes in the world, but only about 50 major ones.
Most of them, including the Nasdaq composite and the S&P 500, are value-weighted. That means stocks with larger market values account for a larger share of the index’s performance.
In addition to these broad-based indexes, there are many less prominent ones. Many of those emphasize a niche by tracking stocks of companies in specific industries like energy or finance.
Do these milestones matter?
Stock prices move constantly in response to corporate, economic and political news, as well as changes in investor psychology. Because company profits will typically grow gradually over time, the market usually fluctuates in the short term, while increasing in value over the long term.
The DJIA first reached 1,000 in November 1972, and it crossed the 10,000 mark on March 29, 1999. On Jan. 22, 2024, it surpassed 38,000 for the first time. Investors and the media will treat the new record set when it gets to another round number – 40,000 – as a milestone.
The S&P 500 index had never hit 5,000 before. But it had already been breaking records for several weeks.
Because there’s a lot of randomness in financial markets, the significance of round-number milestones is mostly psychological. There is no evidence they portend any further gains.
For example, the Nasdaq composite first hit 5,000 on March 10, 2000, at the end of the dot-com bubble.
The index then plunged by almost 80% by October 2002. It took 15 years – until March 3, 2015 – for it return to 5,000.
By mid-February 2024, the Nasdaq composite was nearing its prior record high of 16,057 set on Nov. 19, 2021.
Index milestones matter to the extent they pique investors’ attention and boost market sentiment.
Investors afflicted with a fear of missing out may then invest more in stocks, pushing stock prices to new highs. Chasing after stock trends may destabilize markets by moving prices away from their underlying values.
When a stock index passes a new milestone, investors become more aware of their growing portfolios. Feeling richer can lead them to spend more.
This is called the wealth effect. Many economists believe that the consumption boost that arises in response to a buoyant stock market can make the economy stronger.
Is there a best stock index to follow?
Not really. They all measure somewhat different things and have their own quirks.
For example, the S&P 500 tracks many different industries. However, because it is value-weighted, it’s heavily influenced by only seven stocks with very large market values.
Known as the “Magnificent Seven,” shares in Amazon, Apple, Alphabet, Meta, Microsoft, Nvidia and Tesla now account for over one-fourth of the S&P 500’s value. Nearly all are in the tech sector, and they played a big role in pushing the S&P across the 5,000 mark.
This makes the index more concentrated on a single sector than it appears.
But if you check out several stock indexes rather than just one, you’ll get a good sense of how the market is doing. If they’re all rising quickly or breaking records, that’s a clear sign that the market as a whole is gaining.
Sometimes the smartest thing is to not pay too much attention to any of them.
For example, after hitting record highs on Feb. 19, 2020, the S&P 500 plunged by 34% in just 23 trading days due to concerns about what COVID-19 would do to the economy. But the market rebounded, with stock indexes hitting new milestones and notching new highs by the end of that year.
Panicking in response to short-term market swings would have made investors more likely to sell off their investments in too big a hurry – a move they might have later regretted. This is why I believe advice from the immensely successful investor and fan of stock index funds Warren Buffett is worth heeding.
Buffett, whose stock-selecting prowess has made him one of the world’s 10 richest people, likes to say “Don’t watch the market closely.”
If you’re reading this because stock prices are falling and you’re wondering if you should be worried about that, consider something else Buffett has said: “The light can at any time go from green to red without pausing at yellow.”
And the opposite is true as well.
Alexander Kurov does not work for, consult, own shares in or receive funding from any company or organization that would benefit from this article, and has disclosed no relevant affiliations beyond their academic appointment.
dow jones sp 500 nasdaq stocks covid-19-
Uncategorized2 weeks ago
All Of The Elements Are In Place For An Economic Crisis Of Staggering Proportions
-
Uncategorized1 month ago
Cathie Wood sells a major tech stock (again)
-
Uncategorized3 weeks ago
California Counties Could Be Forced To Pay $300 Million To Cover COVID-Era Program
-
Uncategorized2 weeks ago
Apparel Retailer Express Moving Toward Bankruptcy
-
Uncategorized3 weeks ago
Industrial Production Decreased 0.1% in January
-
International1 month ago
War Delirium
-
Uncategorized3 weeks ago
RFK Jr: The Wuhan Cover-Up & The Rise Of The Biowarfare-Industrial Complex
-
Uncategorized3 weeks ago
GOP Efforts To Shore Up Election Security In Swing States Face Challenges