How machine learning and artificial intelligence can drive clinical innovation
How machine learning and artificial intelligence can drive clinical innovation
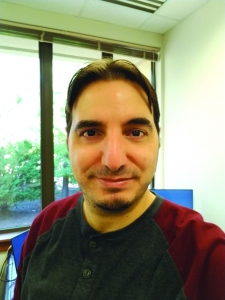
By:
Dr. Basheer Hawwash, Principal Data Scientist
Amanda Coogan, Risk-Based Monitoring Senior Product Manager
Rhonda Roberts, Senior Data Scientist
Remarque Systems Inc.
Everyone knows the terms “machine learning” and “artificial intelligence.” Few can define them, much less explain their inestimable value to clinical trials. So, it’s not surprising that, despite their ability to minimize risk, improve safety, condense timelines, and save costs, these technology tools are not widely used by the clinical trial industry.
There are lots of reasons for resistance: It seems complicated. Those who are not statistically savvy may find the thought of algorithms overwhelming. Adopting new technology requires a change in the status quo.
Yet, there are more compelling reasons for adoption – especially as the global pandemic has accelerated a trend toward patient-centricity and decentralized trials, and an accompanying need for remote monitoring.
Machine learning vs. artificial intelligence. What’s the difference?
Let’s start by understanding what the two terms mean. While many people seem to use them interchangeably, they are distinct: machine learning can be used independently or to inform artificial intelligence; artificial intelligence cannot happen without machine learning.
Machine learning is a series of algorithms that analyze data in various ways. These algorithms search for patterns and trends, which can then be used to make more informed decisions. Supervised machine learning starts with a specific type of data – for instance, a particular adverse event. By analyzing the records of all the patients who have had that specific adverse event, the algorithm can predict whether a new patient is also likely to suffer from it. Conversely, unsupervised machine learning applies analysis such as clustering to a group of data; the algorithm sorts the data into groups which researchers can then examine more closely to discern similarities they may not have considered previously.
In either case, artificial intelligence applies those data insights to mimic human problem-solving behavior. Speech recognition, self-driving cars, even forms that auto-populate all exist because of artificial intelligence. In each case, it is the vast amounts of data that have been ingested and analyzed by machine learning that make the artificial intelligence application possible.
Physicians, for instance, can use a combination of machine learning and artificial intelligence to enhance diagnostic abilities. In this way, given a set of data, machine learning tools can analyze images to find patterns of chronic obstructive pulmonary disease (COPD); artificial intelligence may be able to further identify that some patients have idiopathic pulmonary fibrosis (IPF) as well as COPD, something their physicians may neither have thought to look for, nor found unaided.
Now, researchers are harnessing both machine learning and artificial intelligence in their clinical trial work, introducing new efficiencies while enhancing patient safety and trial outcomes.
The case of the missing data
Data is at the core of every clinical trial. If those data are not complete, then researchers are proceeding on false assumptions, which can jeopardize patient safety – and even the entire trial.
Traditionally, researchers have guarded against this possibility by doing painstaking manual verification, examining every data point in the electronic data capture system to ensure that it is both accurate and complete. More automated systems may provide reports that researchers can look through – but that still requires a lot of human involvement. The reports are static and must be reviewed on an ongoing basis – and every review has the potential for human error.
Using machine learning, this process happens continually in the background throughout the trial, automatically notifying researchers when data are missing. This can make a material difference in a trial’s management and outcomes.
Consider, if you will, a study in which patients are tested for a specific metric every two weeks. Six weeks into the study, 95 percent of the patients show a value for that metric; 5 percent don’t. Those values are missing. The system will alert researchers, enabling them to act promptly to remedy the situation. They may be able to contact the patients in the 5 percent and get their values, or they may need to adjust those patients out of the study. The choice is left to the research team – but because they have the information in near-real time, they have a choice.
As clinical trials move to new models, with greater decentralization and greater reliance on patient-reported data, missing data may become a larger issue. To counteract that possibility, researchers will need to move away from manual methods and embrace both the ease and accuracy of machine-learning-based systems.
The importance of the outlier
In research studies, not every patient – nor even every site – reacts the same way. There are patients whose vital signs are off the charts. Sites with results that are too perfect. Outliers.
Often researchers discover these anomalies deep into the trial, during the process of cleaning the data in preparation for regulatory submission. That may be too late for a patient who is having a serious reaction to a study drug. It also may mean that the patient’s data are not valid and cannot be included in the end analysis. Caught earlier, there would be the possibility of a course correction. The patient might have been able to stay in the study, to continue to provide data; alternatively, they could be removed promptly along with their associated data.
Again, machine learning simplifies the process. By running an algorithm that continually searches for outliers, those irregularities are instantly identified. Researchers can then quickly drill down to ascertain whether there is an issue and, if so, determine an appropriate response.
Of course, an anomaly doesn’t necessarily flag a safety issue. In a recent case, one of the primary endpoints involved a six-minute walk test. One site showed strikingly different results; as it happened, they were using a different measurement gauge, something that would have skewed the study results, but, having been flagged, was easily modified.
In another case, all the patients at a site were rated with maximum quality of life scores – and all their blood pressure readings were whole numbers. Machine learning algorithms flagged these results because they varied dramatically from the readings at the other sites. On examination, researchers found that the site was submitting fraudulent reports. While that was disturbing to learn, the knowledge gave the trial team power to act, before the entire study was rendered invalid.
A changing landscape demands a changing approach
As quality management is increasingly focusing on risk-based strategies, harnessing machine learning algorithms simplifies and strengthens the process. Setting parameters based on study endpoints and study-specific risks, machine learning systems can run in the background throughout a study, providing alerts and triggers to help researchers avoid risks.
The need for such risk-based monitoring has accelerated in response to the COVID-19 pandemic. With both researchers and patients unable or unwilling to visit sites, studies have rapidly become decentralized. This has coincided with the emergence and growing importance of patient-centricity and further propelled the rise of remote monitoring. Processes are being forced online. Manual methods are increasingly insufficient – and automated methods that incorporate machine learning and artificial intelligence are gaining primacy.
Marrying in-depth statistical thinking with critical analysis
The trend towards electronic systems does not replace either the need for or the value of clinical trial monitors and other research personnel; they are simply able to do their jobs more effectively. A machine-learning-based system runs unique algorithms, each analyzing data in a different way to produce visualizations, alerts, or workflows, which CROs and sponsors can use to improve patient safety and trial efficiency. Each algorithm is tailored to the specific trial, keyed to endpoints, known risks, or other relevant factors. While the algorithms offer guidance, the platform does not make any changes to the data or the trial process; it merely alerts researchers to examine the data and determine whether a flagged value is clinically significant. Trial personnel are relieved of much tedious, reproducible, manual work, and are able to use their qualifications to advance the trial in other meaningful ways.
The imperative to embrace change
Machine learning and artificial intelligence have long been buzzwords in the clinical trial industry – yet these technologies have only haltingly been put to use. It’s time for that pendulum to swing. We can move more quickly and more precisely than manual data verification, and data cleaning allow. We can work more efficiently if we harness data to drive trial performance rather than simply to prove that the study endpoints were achieved. We can operate more safely if we are programmed for risk management from the outset. All this can be achieved easily, with the application of machine learning and artificial intelligence. Now is the time to move forward.
International
TikTok Ban Obscures Chinese Stock Gold Rush
No one wants to invest in China right now. The country’s stock market is teetering on the brink of collapse. And it is about to lose its biggest foothold…
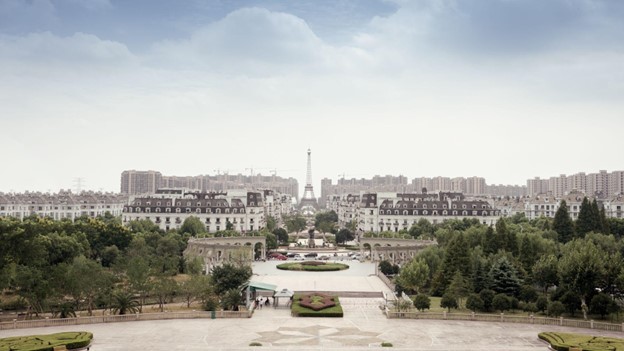
No one wants to invest in China right now.
The country’s stock market is teetering on the brink of collapse.
And it is about to lose its biggest foothold in America — TikTok.
Yet, beneath its crumbling economy, military weather balloons and blatant propaganda tools lie some epic opportunities…
…if you have the stomach and the knowledge.
Because as Jim Woods wrote in his newsletter last month:
“China has been so battered for so long, that there is a lot of deep value here for the ‘blood in the ‘’red’’ streets’ investors.”
And boy was he right.
However, this battle-tested veteran didn’t recommend buying individual Chinese stocks.
He was more interested in the exchange-traded funds (ETFs) like the CHIQ.
And here’s why…
Predictable Manipulation
China’s heavy-handed approach creates gaping economic inefficiencies.
When markets falter, President Xi calls on his “national team” to prop up prices.
$17 billion flowed into index-tracking funds in January as the Hang Sang fell over 13% while the CSI dropped over 7%.
Jim Woods saw this coming from a mile away.
In late February, he highlighted the Chinese ETF CHIQ in late February, which has rallied rather nicely since then.
This ETF focuses on the Chinese consumer, a recent passion project for the central government.
You see, around 2018, when President Xi decided to smother his own economy, notable shifts were already taking place.
The once burgeoning retail market had slowed markedly. Developers left cities abandoned, including weird copies of Paris (Tianducheng) and England.
Source: Shutterstock
So, Xi and co. shifted the focus to the consumer… which went terribly.
For starters, a lot of the consumer wealth was tied up in real estate.
Then you had a growing population of unemployed younger adults who didn’t have any money to spend.
Once the pandemic hit, everything collapsed.
That’s why it took China far longer to recover even a sliver of its former economy.
While it’s not the growth engine of the early 2000s, the old girl still has some life left in it.
As Jim pointed out, China’s consumer spending rebounded nicely in Q4 2023.
Source: National Bureau of Statistics of China
Combined with looser central bank policy, it was only a matter of time before Chinese stocks caught a lift.
The resurgence may be largely tied to China’s desire to travel. After all, its people have been cooped up longer than any other country.
But make no mistake, this doesn’t make China a long-term investment.
Beyond what most people understand about China’s politics, there’s a little-known fact about how they treat foreign investors.
Money in. Nothing out.
When we buy a stock, we’re taking partial ownership in that company. This entitles us to a portion of the profits (or assets).
That doesn’t happen with Chinese companies.
American depository receipts (ADRs) aren’t actual shares of a company. It’s a note that the intermediary ties to shares of the company they own overseas.
So, we can only own Chinese companies indirectly.
But there’s another key feature you probably weren’t aware of.
Many of the Chinese companies we, as Americans invest in, don’t pay dividends. In fact, a much smaller percentage of Chinese companies pay any dividends.
Alibaba is a perfect example.
Despite generating billions of dollars in cash every year, it doesn’t pay dividends.
What do its managers do with the money?
Other than squirreling away $80 billion on its balance sheets, they do share buybacks.
Plenty of investors will tell you that’s even better than dividends.
But you have no legal ownership rights in China. So, what is that ADR in reality?
We’d argue nothing but paper profits at best, and air at worst.
That’s why it’s flat-out dangerous to own shares of individual Chinese companies long-term.
Any one of them can be nationalized at any moment.
Chinese ETFs reduce that risk through diversification, similar to junk bond funds.
Short of an all-out ban, like between the United States and Russia, the majority of the ETF holdings should remain intact.
Opportunistic Investing
If China is so unstable, and capable of changing at a moment’s notice, how can investors uncover pockets of value?
As Jim showed with his ETF selection, you can have some sector or thematic idea so long as you have the data to support it.
China, like any large institution, isn’t going to change its broad economic policies overnight.
As long as you study the general movements of the government, you can steer clear of the catastrophic zones and towards the diamond caves.
Because when things look THIS bad, you know the opportunities are even juicier.
But rather than try to run this maze solo, take this opportunity to check out Jim Woods’ latest report on China.
In it, he details the broad economic themes driving the Chinese government, and how to exploit them for gain.
Click here to explore Jim Woods’ report.
The post TikTok Ban Obscures Chinese Stock Gold Rush appeared first on Stock Investor.
stocks pandemic real estate etf consumer spending gold russia chinaInternational
The Great Escape… of UK Unemployment Reporting
https://bondvigilantes.com/wp-content/uploads/2024/03/1-the-great-escape-of-uk-unemployment-reporting-1024×576.pngThe Bank of England Monetary Policy Committee…
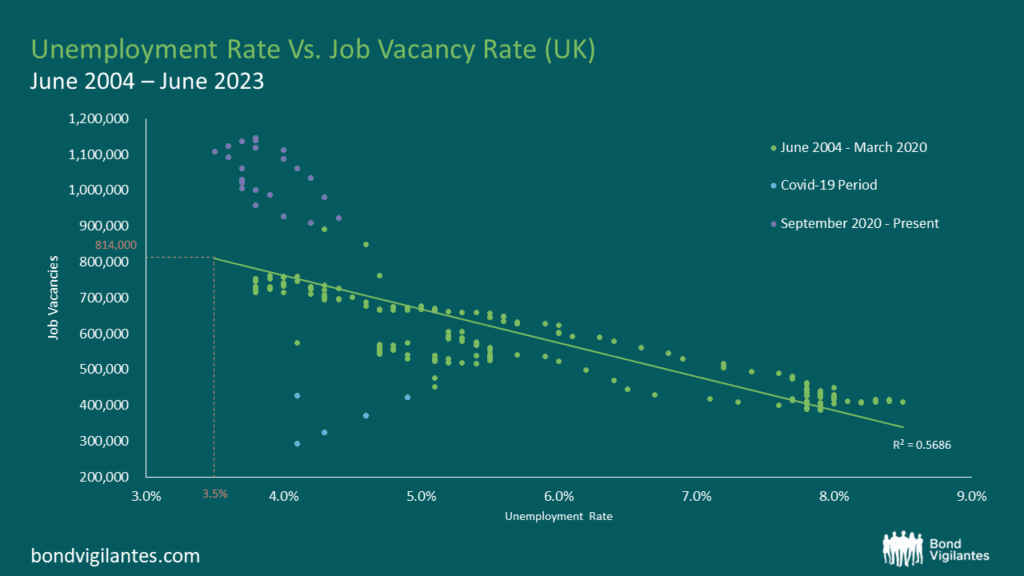
The Bank of England Monetary Policy Committee potentially has a problem: it requires data to make its labour market forecasts and assessments, but the unemployment statistics have become increasingly unreliable. This is because the Labour Force Survey participation rate (on which the unemployment figures are based) has fallen below 50% since 2018 and has been as low as 15% recently[1]. What is the solution to this difficult measurement problem? An answer can be found in the classic war film, The Great Escape.
In 1943, the Escape Committee of Stalag Luft III was tasked with digging a tunnel to freedom. Unfortunately, they had a problem. They needed to measure the distance between one of the prisoner’s huts and the forest beyond the prison perimeter, but they had no reliable tools to measure this critical variable. Fortunately they had two mathematicians within the group who came up with a method to gauge the distance to the forest so that the tunnel would be long enough to ensure escape without detection. The idea was to eyeball the distance using a 20 foot tree for scale (the tree was the one ‘accurate’ measurement around which they could work with). They got individual prisoners to gauge the distance from the hut to the tree and then averaged all of the estimates. The critical distance measure was therefore the average of a large sample size of guesstimates. Fortunately, it more or less worked. Happily, modern economists have an equivalent to rely on in the area of unemployment. Their version of the Stalag Luft III tree strategy is something called the Beveridge Curve.
The Beveridge Curve is simply an observed relationship between an economy’s unemployment rate and its job vacancy rate at the same point in time. An excellent exposition can be found in the Bond Vigilantes archive[2]. When you plot the two variables against one another over a given period, the data points disclose a curve. This curve shows us that when unemployment increases, job vacancies decrease and vice versa. I have plotted the current curve below using the available data from the Office for National Statistics (ONS)[3]. The bottom left quadrant of the graph (the blue dots) relate to the Covid-19 era and the top left quadrant (the purple dots) represent the last 2 years’ worth of data. The green dots represent the remaining data from July 2004 to June 2023.
Source: Office for National Statistics, Dataset JP9Z & UNEM

Source: Office for National Statistics, Dataset JP9Z & UNEM
From these charts and new data from the ONS, we can observe that in the UK, the level of unemployment is increasing and that the job vacancy rate is decreasing. At face value, this suggests that current Bank of England monetary policy is working and that the inflation rate is slowing as the economy cools. One could argue that we are on track for a reasonably soft landing. Nothing new so far.
Things become more interesting when we consider the Beveridge Curve in conjunction with the most recent job vacancy data. We are told that there are now 814,000 job vacancies as of the 31st December 2023[4]. Ordinarily, we would use the curve and clearly be able to extrapolate from the Job Vacancy data what our Unemployment figure might be. However, we also know that the current unemployment data is unreliable, which makes this harder. Using our model inclusive of data oddities, we could extrapolate that with 814,000 job vacancies, we might expect an unemployment rate of around 3.5%. Yet, we know that our unemployment figures are unreliable so the question therefore is, how big an increase in unemployment are we likely to see given what we know about job vacancies?
In order to estimate the magnitude of the rise in unemployment, we need to look further afield. If we study the levels of economic inactivity in the UK, we can observe that they have remained stationary at 22%[5] for the last decade. We can also see that the population of the UK has risen over the same period by around 5.91%[6]. Further, we know that the Labour Force Survey (LFS) samples 40,000 households per quarter to obtain its data, but of late has had a response rate of only 15% (6,000 households). Therefore a critical question for policy makers is what is happening with the 85%, the non-responders?
Given the small sample size, it is entirely possible that the LFS suffered survey bias that is being erroneously weighted away. In other words, the LFS compensates for the paucity of response data by accessing other regional population statistics as a legitimate part of their methodology. The problems of non-responders are being addressed in upcoming LFS releases but for the time being, the data is not as clear as it ought to be. With such a small sample size, it seems possible – indeed probable – that unemployment levels are being underreported. This would explain why the current unemployment rate of 3.8%[7] is dramatically lower than the historic average of 6.7% (1971-2023). We see further evidence for this in the forecasts of the UK’s unemployment rate on Bloomberg which have been consistently above the actual levels for the last few published data points. So whilst the published headline figures might be looking reasonable, the underlying story looks like it could be hiding something more sinister.
Through it all, the Beveridge Curve remains a reasonable template. Job vacancies are definitely falling, so we should expect to see unemployment rising. Like the Stalag Luft III measurement solution, the Beveridge Curve offers a constructive way out of our present statistical dilemma. That being said, analogies can only be taken so far. Unfortunately for the inmates of Stalag Luft III, the calculation didn’t quite work and the tunnel came up short. No one actually made a Great Escape. What does this mean for UK unemployment data? Time may tell.
[1] The UK’s ‘official’ labour data is becoming a nonsense (harvard.edu)
[2] https://bondvigilantes.com/blog/2013/11/a-shifting-beveridge-curve-does-the-us-have-a-long-term-structural-unemployment-problem/
[3] Unemployment – Office for National Statistics (ons.gov.uk)
[4] https://www.ons.gov.uk/employmentandlabourmarket/peopleinwork/employmentandemployeetypes/timeseries/jp9z/unem
[5] https://www.ethnicity-facts-figures.service.gov.uk/work-pay-and-benefits/unemployment-and-economic-inactivity/economic-inactivity/latest/#:~:text=data%20shows%20that%3A-,22%25%20of%20working%20age%20people%20in%20England%2C%20Scotland%20and%20Wales,for%20a%20job)%20in%202022
[6] https://www.ons.gov.uk/peoplepopulationandcommunity/populationandmigration/populationestimates/bulletins/annualmidyearpopulationestimates/mid2021
[7] https://www.ons.gov.uk/employmentandlabourmarket/peoplenotinwork/unemployment
unemployment covid-19 monetary policy unemployment ukInternational
Germany Is Running Out Of Money And Debt Levels Are Exploding, Finance Minister Warns
Germany Is Running Out Of Money And Debt Levels Are Exploding, Finance Minister Warns
By John Cody of Remix News
German Finance Minister…
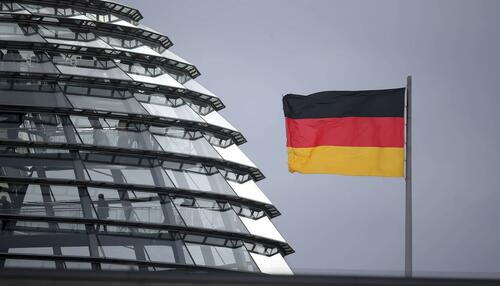
By John Cody of Remix News
German Finance Minister Christian Lindner is warning his own government that state finances are quickly growing out of hand, and the government needs to change course and implement austerity measures. However, the dispute over spending is only expected to escalate, with budget shortfalls causing open clashes among the three-way left-liberal coalition running the country.
With negotiations kicking off for the 2025 budget, much is at stake. However, the picture has been complicated after the country’s top court ruled that the government could not shift €60 billion in money earmarked for the coronavirus crisis to other areas of the budget, with the court noting that the move was unconstitutional.
Since then, the government has been in crisis mode, and sought to cut the budget in a number of areas, including against the country’s farmers. Those cuts already sparked mass protests, showcasing how delicate the situation remains for the government.

Lindner, whose party has taken a beating in the polls, is desperate to create some distance from his coalition partners and save his party from electoral disaster. The finance minster says the financial picture facing Germany is dire, and that the budget shortfall will only grow in the coming years if measures are not taken to rein in spending.
“In an unfavorable scenario, the increasing financing deficits lead to an increase in debt in relation to economic output to around 345 percent in the long term,” reads the Sustainability Report released by his office. “In a favorable scenario, the rate will rise to around 140 percent of gross domestic product by 2070.”
Under EU law, Germany has limited its debt levels to 60 percent of economic output, which requires dramatic savings. A huge factor is Germany’s rapidly aging population, with a debt explosion on the horizon as more and more citizens head into retirement while tax revenues shrink and the social welfare system grows — in part due to the country’s exploding immigrant population.
Lindner’s partners, the Greens and Social Democrats (SPD), are loath to cut spending further, as this will harm their electoral chances. In fact, Labor Minister Hubertus Heil is pushing for a new pension package that will add billions to the country’s debt, which remarkably, Lindner also supports.
Continue reading at rmx.news
-
Uncategorized4 weeks ago
All Of The Elements Are In Place For An Economic Crisis Of Staggering Proportions
-
International1 week ago
EyePoint poaches medical chief from Apellis; Sandoz CFO, longtime BioNTech exec to retire
-
Spread & Containment5 days ago
IFM’s Hat Trick and Reflections On Option-To-Buy M&A
-
Uncategorized1 month ago
California Counties Could Be Forced To Pay $300 Million To Cover COVID-Era Program
-
Uncategorized3 weeks ago
Apparel Retailer Express Moving Toward Bankruptcy
-
Uncategorized1 month ago
Industrial Production Decreased 0.1% in January
-
International1 week ago
Walmart launches clever answer to Target’s new membership program
-
Uncategorized1 month ago
RFK Jr: The Wuhan Cover-Up & The Rise Of The Biowarfare-Industrial Complex